About Me
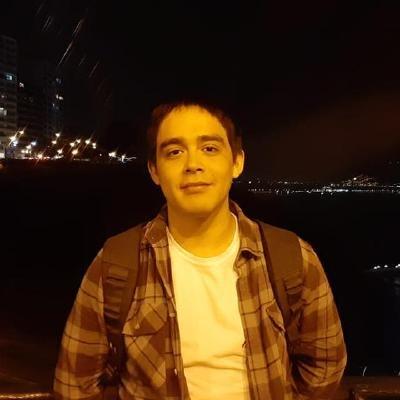
I am a Peruvian geographer/data scientist enrolled in the fantastic Erasmus+ Copernicus Master in Digital Earth program. I work on global EO problems and try to solve them using GEE/Python/R (and somethimes a bit of C++). In my spare time, I teach coding to anyone who needs it and collaborate on a variety of open-source projects. If you want to learn more about me, please see my cv.
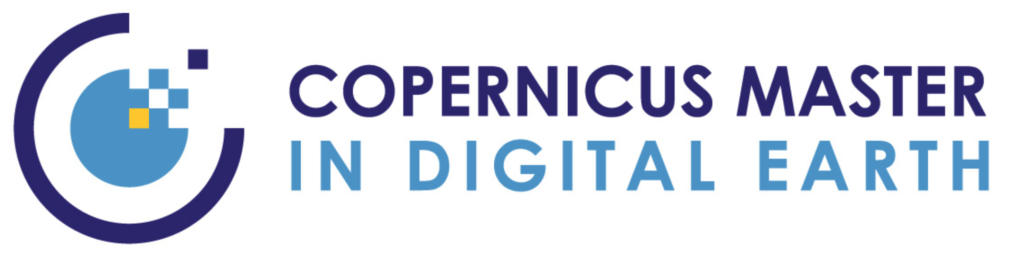
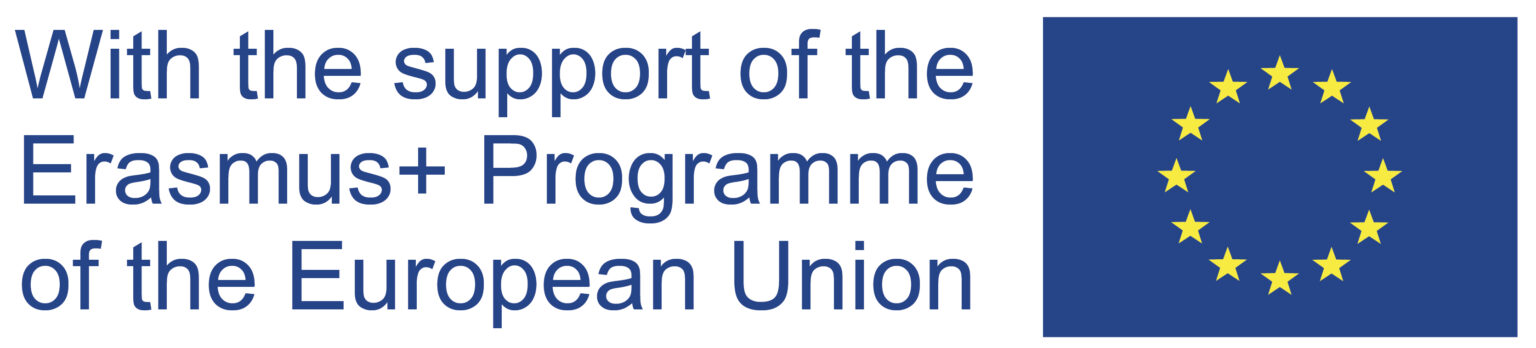
Research Interest
Publications
In chronological order:
- Huerta A., Aybar C., Imfeld N., Correa K., Felipe-Obando O., and Lavado-Casimiro W. An updated high-resolution gridded dataset of daily air temperature for Peru, PISCOt. In preparation.
- Montero D., Aybar C., Mahecha M., Wienek S. spectral: Awesome Spectral Indices deployed via the Google Earth Engine JavaScript API. Submitted.
- Montero D., Aybar C., Mahecha M., Martinuzzi F., Söchting M., Wienek S. Awesome Spectral Indices: an open-source ecosystem for handling spectral indices in Earth Systems research. Submitted.
- Aybar, C., Ysuhuaylas L., Loja J., Gonzales K., Herrera F., Bautista L., Yali R., Flores A., Diaz L. Cuenca N., Prudencio F., LLactayo V., Montero D., Sudmanns M., Tiede D., Mateo-García G., Gómez-Chova L. CloudSEN12 - a global dataset for semantic understanding of cloud and cloud shadow in satellite imagery. In preparation.
- Fernandez-Palomino, C.A., Hattermann, F.F., Krysanova, V., Lobanova, A., Vega-Jácome, F., Lavado, W., Santini, W., Aybar, C. and Bronstert, A., 2022. A Novel High-Resolution Gridded Precipitation Dataset for Peruvian and Ecuadorian Watersheds: Development and Hydrological Evaluation. Journal of Hydrometeorology, 23(3), pp.309-336.
- Aybar, C., Wu, Q., Bautista, L., Yali, R., & Barja, A. (2020). rgee: An R package for interacting with Google Earth Engine. Journal of Open Source Software, 5(51), 2272.
- Aybar, C., Fernández, C., Huerta, A., Lavado, W., Vega, F. and Felipe-Obando, O., 2020. Construction of a high-resolution gridded rainfall dataset for Peru from 1981 to the present day. Hydrological Sciences Journal, 65(5), pp.770-785.
- Andrade, M.F., Moreno, I., Calle, J.M., Ticona, L., Blacutt, L., Lavado-Casimiro, W., Sabino, E., Huerta, A., Aybar, C., Hunziker, S. and Brönnimann, S., 2018. Atlas-Clima y eventos extremos del Altiplano Central perú-boliviano.